One frequently-ask question from Metascape users is how to submit a customized background gene list for enrichment analysis. The complete proteome is used as the default background in Metascape, however, this behavior can be overwritten.
_BACKGROUND at Gene List Submission
Metascape can take multiple gene lists as input. For example, in the multi-list Excel upload format, each column contains a gene list. If a gene list is named “_BACKGROUND” (Figure 1), it will be treated as a special content. This works regardless of how many gene lists you intend to analyze (i.e., applicable to a single foreground gene list). Do not worry about whether the foreground genes should be included or excluded in your _BACKGROUND list, as Metascape always append all foreground genes onto the _BACKGROUND list.
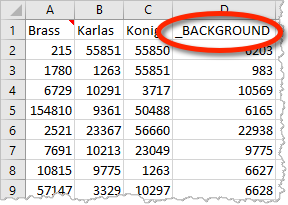
Upon file submission, Metascape shows all the foreground gene lists (Figure 2). Notice that although we uploaded 4 gene lists including “_BACKGROUND” (Figure 1), “_BACKGROUND” list is invisible in this view, as Metascape knows it has a special mission.
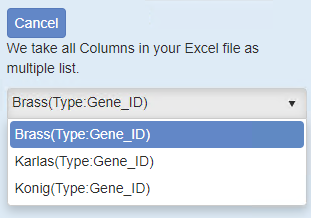
Submit Background in Custom Analysis
You can also define or overwrite background list during Custom Analysis. Before you trigger the “Enrichment Analysis”, click on “Change” button to define/modify background genes.
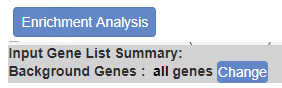
In the popup window, paste in background genes in any identifier type supported by Metascape (such as Symbol, RefSeq, Ensembl, etc.), then click “Submit” and then “Close” the dialog. In Figure 4 we only submit 17 test genes as the background for a 121-gene foreground single list. Metascape automatically identifies and appends 119 foreground genes missed from our pasted list, so that final size of the background gene list is 136. (This is just an example, the list of background genes should be much more in real cases). If you want to modify the existing background list, simply update the content in the text area. If you want to use the default whole-proteome as the background list, click on “Remove” and then “Close” to resort to the default behavior.
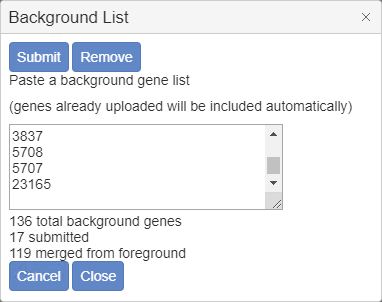
Background Gene List is Ill Defined
Unquestionably background gene list alters the statistical significance (p-values). Background correction becomes critical, when experiments were conducted using a functionally-biased gene collection (such as a kinases siRNA library, a transmembrane CRIPR library, or a bias design due to the constrain of experimental throughput). This induces a technical bias, as all hits identified from a kinase library will be all kinase. We will naturally see enrichments of kinase-related functions, when the whole-proteome is mistakenly used as the background and such enrichments are probably false positives. For instance, Timmons et al. discovered “acetylation” is highly enriched on Affymetrix’s U133 GeneChip (p < \(10^{-51}\)) simply due to its composition [1]. We strongly advise users to provide background list when such custom profiling platform is used.
Thanks to technology advancement, whole-genome and whole-proteome OMICs technologies are in routine use nowadays. Design-related technology bias is less of a concern now, but technology bias remains a challenge. For instance, in RNA-Seq, not all mRNA will be sequenced with equal efficiency and reliability, which is another form of technical bias that is much harder to assess.
Furthermore, the biological system to be studied are biased to begin with. When study a brain sample, many genes are not expressed, therefore, will never had any chance to land on the hit list. Such genes should have been excluded from the background list, however, what genes are expressed in the system often cannot be predetermined. This biological bias has a significant effect and is very hard to taken into account.
To complicated matter further, background gene list is not the only factor affecting p-value calculation. Many subjective factors affecting our definition of the foreground hit list, such as fold change and p-value cutoffs users apply to define differentially expressed hit candidates. The ontology database used for enrichment analysis has intrinsic redundancy, therefore, p-values would need to go through a multi-test correction process. Unfortunately how to carry out such correction remains an open question (see out previous blog on q-value calculation).
Altogether, the accurate determination of enrichment p-values are nearly impossible due to following reasons: (1) true background genes are poorly defined; (2) the list of true foreground genes is subjective; (3) how to perform multi-test correction to p-values remain open. Although Timmons et al. and many bioinformaticians are absolutely right about the need to provide background gene list in enrichment studies, unfortunately it is not an actionable requirement in the majority circumstance.
Final Thoughts
We share similar views as DAVID that in most OMIC studies, although the exact list of background genes affects the magnitudes of p-values, it should only have limited effects on the relative ranking of the enriched terms [2]. We also agree with Timmons et al. that users should avoid interpreting enrichment terms that have only marginal significance around \(10^{-3}\) or \(10^{-4}\), as they might simply become statistically insignificant, if the list of background genes change or a different multi-test correction algorithm is used. Metascape uses \(10^{-2}\) as the default p-value threshold, because a more stringent cutoff might filter out legit enriched terms in studies that are relatively noisier and more difficult to characterize due to their biological nature. We believe the right attitude on the functional enrichment analysis is to treat it as a guidance to filter and rank pathways and processes, but not to religiously believe in the absolute numbers. If the terms of interest have very good p-values (< \(10^{-6}\)) and your system is studied with unbiased OMIC platforms, what background to use and what multi-test correction to apply should not be a concern. Providing enrichment p-values should be sufficient for publication needs, but be prepared to supply q-values, if referees demand more.
Reference
- Timmons JA, Szkop KJ, Gallagher IJ. Multiple sources of bias confound functional enrichment analysis of global -omics data. Genome Biol. 2015 16:186.
- Huang da W, Sherman BT, Lempicki RA. Systematic and integrative analysis of large gene lists using DAVID bioinformatics resources. Nat Protoc. 2009;4(1):44-57.
Visualize Networks Online and Offline
Metascape provides intuitive visualizations for either pathway enrichment networks or protein-protein interaction networks. By default, we do not display labels for the nodes within the .png and .pdf outputs, because displaying them will make both networks and labels unreadable (Figure 1).
In order to interpret the network, we recommend users to open the network .cys file within Cytoscape application. For those users who do not have Cytoscape installed or just want a quick examination of the networks, Metascape now provides an easy alternative to display networks within the browser.
Visualization within Browser
On the Analysis Report web page. Users can click on the Web icon (Figure 2) to open an interactive browser page. The network is displayed using CytoscapeJS, a simplified Cytoscape web client.
In the web view, one can use mouse scroll to zoom in/out. The labels for the nodes can be displayed when they are mouse selected (Figure 3). The application seems to be buggy (at least when the labels have not been displayed yet), if you do not see labels, try to click on the background to unselect nodes first, then mouse select the nodes again.
We also included CytoscapeJS application in the Zip package. The AnalysisReport.html file contained with the zip file provides the same Web icon that can lead to the similar web view (works without Internet connection).
Cytoscape AutoAnnotate
Better visualization options are available by opening the .cys session file within the Cytoscape application. AutoAnnotate is a Cytoscape app preinstalled in Cytoscape (if not, upgrade your Cytoscape or use menu Apps > App Manager to install).
To run AutoAnnotate, choose menu Apps > AutoAnnotate > New Annotation Set … (Figure 4).
Configure the Advanced options like the following (Figure 5). This is for the GO enrichment network. There is no need to use AutoAnnotate for protein-protein interaction network, as we display gene symbols for all MCODE networks by default.
The result is shown below (Figure 6). There are settings (shown on the right panel) to adjust colors and font sizes. Notice that AutoAnnotate nicely extracts keywords for each cluster to provide a functional overview. Since the default effect can be rather crowded, AutoAnnotate is not used by Metascape to produce publishable graphics.
If you would like move clusters apart for a clearer view, it is important to use menu Layout > Clear All Edge Bends to unbundle edges first.
Then select nodes and move them to desirable locations:
Then bundle the edges afterwards for a more aesthetic view using menu Layout > Bundle Edges > All Nodes and Edges.
We turn Figure 9 into Figure 10:
If you would like to publish the final graph, use menu File > Export > Network to Image …